Table Of Contents:
Why multimodal data is the new frontier of data science
How multi-dimensional arrays solve frontier data’s problems of scale for life science research teams
Scientific discovery requires a new approach to multimodal data today
If you were searching for a famous treasure, would you rather look in the same locations others had already tried, or try entirely new areas that were ripe for discovery?
This frontier, multimodal data has the potential to revolutionize how we treat cancer and other serious diseases, and we have a moral obligation to pursue this data’s possibility. In this post, we will examine why multimodal data is the new frontier of data science, how multi-dimensional arrays can analyze and manage this frontier data at scale and what multi-dimensional arrays offer different stakeholders inside life sciences orgs.
Why multimodal data is the new frontier of data science
The vast majority of database solutions in life sciences are architected for tabular data. Organized in rows and columns, this tabular data follows the relational model used for everyday data like payroll information, CRM databases and other business recordkeeping. This tabular data approach works great if your data fits easily into a spreadsheet. If, however, you’re trying to process the more than 500,000 genomic samples in a large biobank like UK Biobank, tabular databases lack the performative scale to manage this data effectively.
In short, the scale and complexity of the data life sciences organizations must manage is beyond the reach of traditional databases. This is why we call it frontier data. As the new frontier of data science, this multimodal data is both novel and highly valuable. Here’s what we mean by that:
Novel: This data is without precedent in its scope, and drawn from new sources like genomics and transcriptomics, especially at the single cell or spatial levels.
Valuable: This data embraces the complexity of biology, and holds the key to breakthrough discoveries in science and industry, going beyond simple treatment iterations to finding novel precision medicine for cancer and genetic diseases.
Unstructured, or often semi-structured, large-scale, frontier data is inherently complex. Frontier data stresses the limits of status quo solutions and compute management, so tabular databases simply cannot deliver the scale or performance required to analyze this data. No matter how many data scientists or how much cloud computing you throw at frontier data, it needs a more powerful data structure than tabular databases.
How multi-dimensional arrays solve frontier data’s problems of scale for life science research teams
One of the foundational beliefs of TileDB is that no data is unstructured. This term has often been used as an excuse to ignore multimodal data that doesn’t fit easily into a tabular database. A multi-dimensional array moves past the limits of tables by bringing structure to even the most complex data types like genomics, single cell and imaging in life sciences. Because arrays can flexibly shift their shape based on data input, they can perfectly adapt to all kinds of data, whether tabular or frontier.
This multidimensional array approach is already solving the problems of scale that have held back life sciences organizations from mastering frontier data:
Rady Children’s Institute of Genomic Medicine
processed their VCF samples using multidimensional arrays on Amazon S3, performing analyses that once took days in seven hours.
relied on multidimensional arrays to analyze transcriptomic data from hundreds of millions of single cells to support deep learning models that inform drug development.
used multidimensional arrays to scale complex metadata queries and support unique single-cell access patterns for their accelerated implementations of key tools like differential gene expression.
Drilling down from the organizational level, multidimensional arrays also have a lot to offer individual members of a life sciences research team.
Research scientists
can tackle complex data problems faster and at greater scale than ever before while making their data FAIR and ready for machine learning applications. Sharing frontier data in a multidimensional array also facilitates secure collaboration across teams and organizations and makes data more discoverable for easier access.
Data engineers and bioinformaticians
can build more efficient and secure data infrastructure to process large data queries at scale. Because curating and preparing data is much simpler with a flexible multidimensional array, teams spend less on computing and coding costs.
IT managers
can achieve significant savings in cloud storage and technology spend. Rady Children’s Institute for Genomic Medicine found a 97% cost reduction by modeling VCF data as multidimensional arrays on Amazon S3 compared to their old file-based approach.
Scientific discovery requires a new approach to multimodal data today
We have a moral mandate to change the data status quo. As we explore new ways to treat and cure cancer and genetic diseases, those suffering from these ailments cannot afford to wait. Relational tables have enabled great progress in the past, but the urgency of the present moment requires a new approach. It’s time to embrace the flexibility and scale of multidimensional arrays.
At TileDB, we believe that multidimensional arrays have great promise not only for frontier data but also the everyday data served by traditional databases. Because of their shape-shifting nature, arrays adapt easily and bring structure to any type of data, improving performance and accelerating discovery for modalities ranging from genomics to relational tables. The result is a database that does more than simply help query data—it is a database designed for discovery of deep and meaningful insights.
About the author
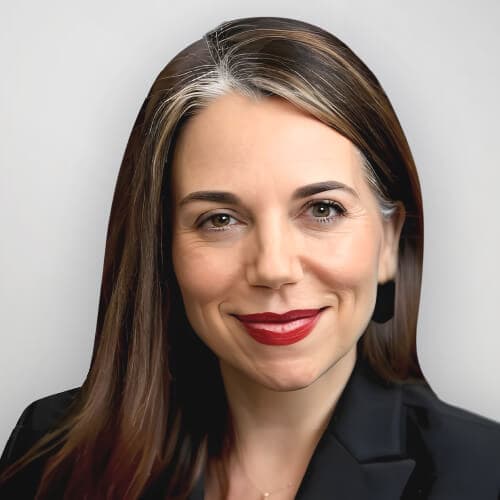
Julie Bryce
Chief Commercial Officer
Julie Bryce leads Go to Market at TileDB. She brings 20 years of experience from roles at Red Hat and Oracle, as well as venture-backed startups. Specializing in complex B2B technology, she launched a fractional Chief Marketing Officer practice in 2018, collaborating closely with 20+ firms, many P/E or VC-backed and founder-led. Before TileDB, Julie led go to market and strategy for an AI/NLP startup, guiding it through the disruptive market entry of generative AI, culminating in a successful Series A funding round. She lives in North Carolina with her partner and two kids. She loves road trips, strong coffee and NW Montana.
Meet the authors
Julie Bryce
Chief Commercial Officer